Geoffroy Oudoumanessah
Statify @ Inria, Grenoble Institute of Neurosciences, CREATIS
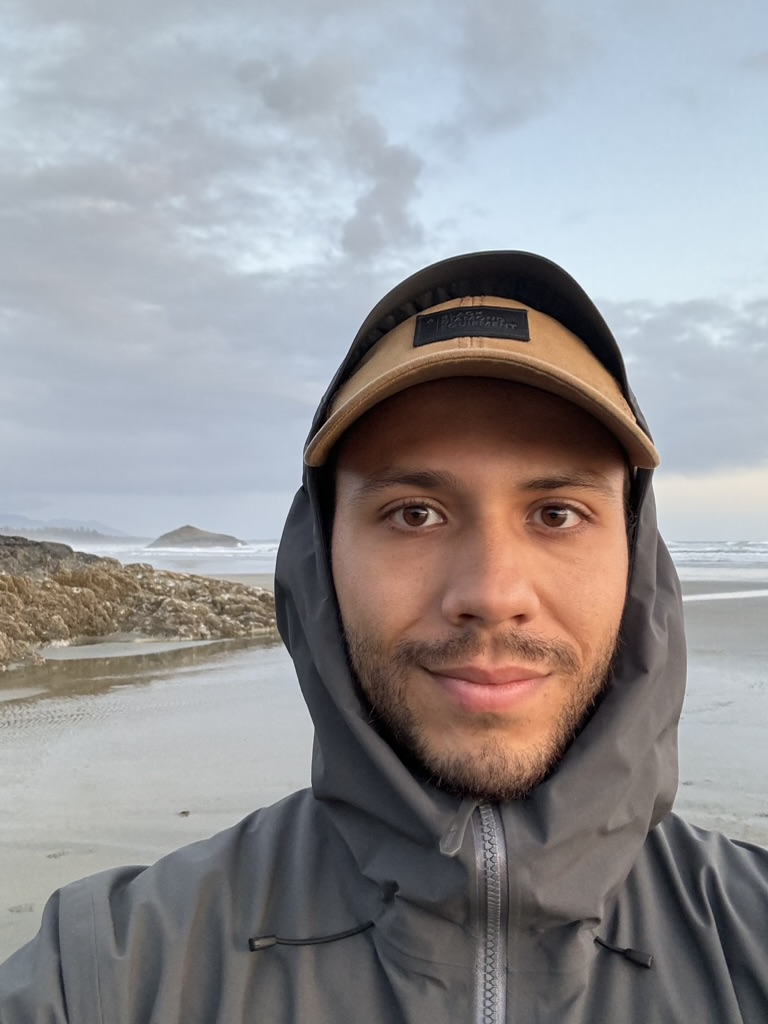
Grenoble, France
I am a last year PhD student in statistics with a deep interest in brain MRI in the STATIFY team at Inria, the Grenoble Institute of Neurosciences and CREATIS. I have an engineering degree in Computer Science and Applied Mathematics at Grenoble-INP Ensimag, and a masterβs degree in Mathematics, Computer Vision & Machine Learning from ENS Paris-Saclay.